Consumers generate different kinds of data whenever they interact with brands, at a rate unlike ever before. If you’ve shopped online from a major retailer, every stage in the customer journey will have been quantified to some degree. The information you leave will be added to larger data sets packed with information from other consumers. Then data engineers will use data analytics platforms to extract actionable insights.
Amazon gathers data on every visitor who scans or purchases from their platform, building profiles aimed at selling you the products it thinks you want.
Data is gold in this increasingly digital world. When used with predictive analytics, companies can identify crucial issues, deliver personalized customer experiences, and make quick strategic decisions for the future of the company.
Today, predictive analytics can be used in various areas of business:
- Marketing – predicting purchase and consumption patterns
- Operations – improving supply chain management
- Sales – predicting income and sales revenues
- Finance – forecasting operational risks
Predictive analytics isn’t something new. Still, many companies are hesitant to invest in it due to its complexity and costs. However, in the age of big data, the landscape of business is evolving along with the technologies that power it. Each year, innovative solutions become less complicated and more affordable.
Here, you will discover why investing in predictive analytics is good for your ecommerce business. Find out which brands have embraced data-driven models and how they’re using them to move their business forward.
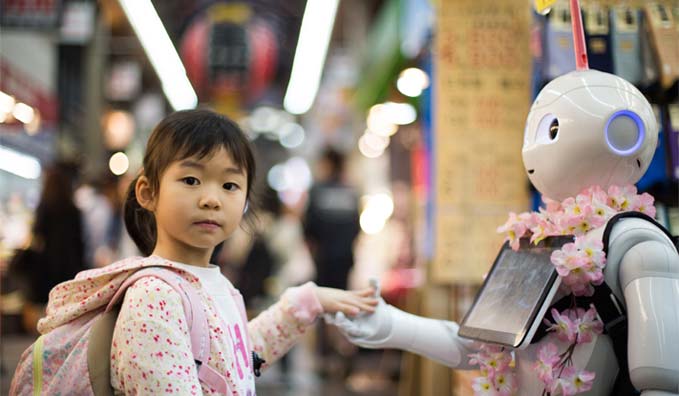
Why do ecommerce businesses need predictive analytics?
The ecommerce industry is one of the largest consumers of data. With huge volumes of data at hand, predictive analytics empowers businesses to make sense of all that information to improve nearly every aspect of business.
Ecommerce brands can use predictive analytics in a number of ways, including:
- Targeted recommendation
- User churn prediction
- Predictive search
- Customer segmentation
- Fraud management
- Price management
- Product inventory optimization
- Business intelligence
For online retailers, investing in predictive analytics will give them a potential edge in this extremely competitive industry. Of course, all models must be tested thoroughly prior to deployment. Keep in mind that for most companies, these data analytics models still need to be overseen by human supervisors.
Predictive analytics applications in ecommerce
Several online retailers are using predictive analytics to understand their consumers and deliver ideal product offerings. Let’s take a closer look at a few e-commerce brands that have adopted data-driven models.
Amazon
Amazon has a recommendation system which determines what their customers want by creating a profile of who they are. The company gathers data on every visitor who scans or purchases from their platform. Based on that information, the system comes up with a profile and offers you products that consumers with similar profiles have purchased before.
Using predictive analytics, Amazon is able to increase its sales and profit margins. At the same time, it enables the retail giant to decrease delivery time and operational costs.
Alibaba
On Singles’ Day in 2017, the Chinese e-commerce giant generated $25 billion in sales. That’s more than Cyber Monday and Black Friday online sales combined. Artificial intelligence and predictive analytics made all of that possible.
Alibaba’s data science lab made a system called the E-Commerce Brain, which predicts consumer needs based on real-time online data. AI capabilities enable the retailer to update its analytics models for each prospect by assessing online activities, browsing history, as well as purchase history.
Netflix
Aside from creating successful films and shows, Netflix’s success is largely due to its ability to determine what their audience wants. The e-commerce giant collects data from their 139 million subscribers, and use data analytics models to understand customer behavior. Using that data, Netflix recommends movies and TV shows based on each user’s preferences.
Netflix has unparalleled customer retention rates due to this. Their recommendation system also accounts for 80% of the content that’s streamed on their platforms.
Stitch Fix
Predictive analytics is the marriage of art and science. Stitch Fix’s online personal styling subscription service is proof of that. For the uninitiated, the company is like a personal stylist. They do all the shopping for their consumers. Clothing pieces and accessories will be mailed to their subscribers, who can then keep items they like, and send back the ones that they don’t.
Needless to say, Stitch Fix takes personalized service to a whole new level. Thanks to predictive analytics, they can use the information they gather from online questionnaires and feedback to pick out what’s best for their consumers.
Methods used in predictive analytics
Each customer has unique online behavior, but ecommerce brands can better understand every prospect and existing customer that visits their online store through predictive analytics. By implementing predictive analytics, risks can be significantly reduced as decisions will be based entirely on verified data.
Predictive analytics encompasses a variety of statistical techniques, including the following:
Data mining
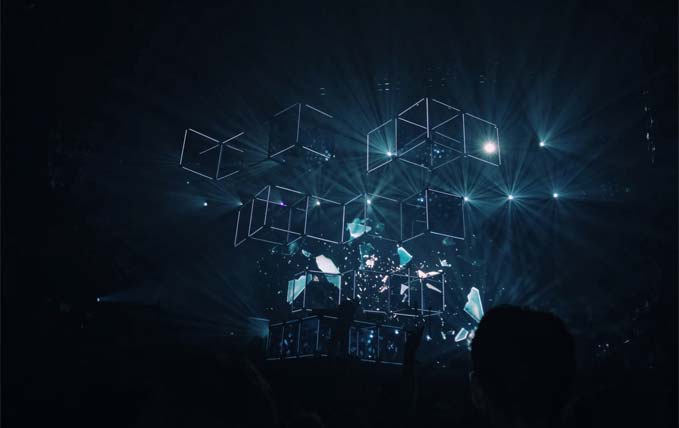
This pertains to the process of collecting and analysing large volumes of data. When mining data, the objective is to identify variables and patterns. All the information is then structured in a comprehensible way. In predictive analytics, data mining is one way of discovering useful trends that the company can harness for future purposes.
Machine learning
Combining machine learning with analytics solutions lets ecommerce brands correlate data from various sources and come up with personalized recommendations for specific segments. Having machine learning capabilities in an analytics model enables businesses of all kinds to assess their target audience’s online behavior. Machine learning can predict behaviors and make decisions with hardly any human supervision.
Artificial intelligence
AI is improving predictive analytics in a multitude of ways. For starters, AI-powered solutions enable ecommerce companies to crunch large quantities of data in real time and deliver accurate predictions.
Deep learning
Made with neural networks, much like a human brain, deep learning capabilities enable analytics models to detect unusual patterns and all kinds of anomalies. This technology can be used to avert catastrophes and prevent system failures, especially in terms of mobile app development.
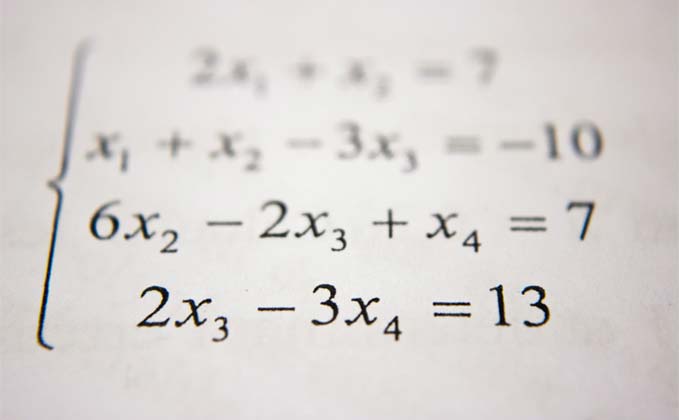
Trends to watch out for in 2019
New and exciting developments in the realm of predictive analytics take shape each year. Let’s take a look at some of the trends that are expected to enhance analytics and insights for ecommerce businesses.
Internet of Things (IoT) analytics
The buzz around the Internet of Things has yet to die down. Perhaps it never will. As IoT devices become increasingly common, there will be more data for businesses to mine. IoT analytics enables entrepreneurs to find valuable insights from huge volumes of data produced by IoT devices.
Augmented analytics
Even in 2018, data scientists and analysts produced most of the qualitative insights for businesses after assessing massive volumes of quantitative data. That’s about to change. These solutions enable data scientists to automate the preparation and analysis of data. Equipped with artificial intelligence and machine learning capabilities, augmented analytics will be able to deliver faster insights.
DataOps
The DataOps methodology is a combination of Agile and DevOps methods. From the collection and preparation of data to analytics, this method can be used to oversee and control data pipelines. In doing so, ecommerce businesses can enhance the accuracy, as well as the speed, of analytics.
Takeaway
Today, the science of predictive analytics has improved significantly and gained more credibility because of some of the best technological breakthroughs in the digital era.
From artificial intelligence to deep learning, these built-in capabilities have enabled predictive analytics models to arm ecommerce businesses of all sizes with usable quantitative data. Implementing predictive analytics allows companies to develop better products, set advantageous pricing strategies, and provide better service. It’s exactly what entrepreneurs need to successfully grow an ecommerce business.